Yufei Zhu
Yufei Zhu Position: Doctoral Student School/office: School of Science and TechnologyEmail: eXVmZWkuemh1O29ydS5zZQ==
Phone: +46 19 303458
Room: T1227
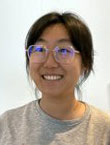
About Yufei Zhu
Welcome to: personal page
I am Yufei Zhu, a doctoral student at the Robot Navigation & Perception Lab, Örebro University in Sweden, under the supervision of Martin Magnusson, with co-supervision by Andrey Rudenko, Tomasz P. Kucner, and Achim J. Lilienthal. I am working on the EU horizon 2020 project DARKO and affiliated with Wallenberg AI, Autonomous Systems and Software Program (WASP).
My research is part of DARKO EU project, which aims to develop agile production robots that are efficient, safe and able to operate in work environments shared with humans. A significant aspect of this environment is the need for robots to be cognizant of human presence and intentions, allowing for smooth and intuitive interactions. To achieve this, the focus is on learning probabilistic representations of human motion patterns, i.e. maps of dynamics (MoDs). By encoding spatio or spatio-temporal patterns of human motion as environmental features, the learned MoDs can be exploited to infer goal locations, constraints, and preferences implicitly, which is crucial for multimodal long-term human motion prediction. Efforts are also directed toward enhancing the efficiency and flexibility of learning these patterns, including online updates with new observations.
Research projects
Active projects
Research groups
Publications
Articles in journals
- Schreiter, T. , Almeida, T. R. d. , Zhu, Y. , Gutiérrez Maestro, E. , Morillo-Mendez, L. , Rudenko, A. , Palmieri, L. , Kucner, T. P. & et al. (2024). THÖR-MAGNI: A large-scale indoor motion capture recording of human movement and robot interaction. The international journal of robotics research. [BibTeX]
- Almeida, T. R. d. , Zhu, Y. , Rudenko, A. , Kucner, T. P. , Stork, J. A. , Magnusson, M. & Lilienthal, A. J. (2024). Trajectory Prediction for Heterogeneous Agents: A Performance Analysis on Small and Imbalanced Datasets. IEEE Robotics and Automation Letters, 9 (7), 6576-6583. [BibTeX]
Conference papers
- Rudenko, A. , Zhu, Y. , Almeida, T. R. d. , Schreiter, T. , Castri, L. , Belotto, N. , Linder, T. , Vaskevicius, N. & et al. (2025). Hierarchical System to Predict Human Motion and Intentions for Efficient and Safe Human-Robot Interaction in Industrial Environments. In: 1st German Robotics Conference. Paper presented at 1st German Robotics Conference, Nuremberg, Germany, March 13-15, 2025. [BibTeX]
- Zhu, Y. , Fan, H. , Rudenko, A. , Magnusson, M. , Schaffernicht, E. & Lilienthal, A. (2024). LaCE-LHMP: Airflow Modelling-Inspired Long-Term Human Motion Prediction By Enhancing Laminar Characteristics in Human Flow. In: 2024 IEEE International Conference on Robotics and Automation (ICRA). Paper presented at IEEE International Conference on Robotics and Automation (ICRA 2024), Yokohama, Japan, May 13-17, 2024. (pp. 11281-11288). IEEE. [BibTeX]
- Zhu, Y. , Rudenko, A. , Kucner, T. , Palmieri, L. , Arras, K. , Lilienthal, A. & Magnusson, M. (2023). CLiFF-LHMP: Using Spatial Dynamics Patterns for Long-Term Human Motion Prediction. In: 2023 IEEE/RSJ International Conference on Intelligent Robots and Systems (IROS), 01-05 October 2023, Detroit, MI, USA. Paper presented at 2023 IEEE/RSJ International Conference on Intelligent Robots and Systems (IROS 2023), Detroit, MI, USA, October 1-5, 2023. (pp. 3795-3802). IEEE. [BibTeX]
- Almeida, T. , Rudenko, A. , Schreiter, T. , Zhu, Y. , Gutiérrez Maestro, E. , Morillo-Mendez, L. , Kucner, T. P. , Martinez Mozos, O. & et al. (2023). THÖR-Magni: Comparative Analysis of Deep Learning Models for Role-Conditioned Human Motion Prediction. In: 2023 IEEE/CVF International Conference on Computer Vision Workshops (ICCVW). Paper presented at IEEE/CVF International Conference on Computer Vision (ICCV) Workshops, Paris, France, October 2-6, 2023. (pp. 2192-2201). IEEE. [BibTeX]
- Schreiter, T. , Almeida, T. R. d. , Zhu, Y. , Gutiérrez Maestro, E. , Morillo-Mendez, L. , Rudenko, A. , Kucner, T. P. , Martinez Mozos, O. & et al. (2022). The Magni Human Motion Dataset: Accurate, Complex, Multi-Modal, Natural, Semantically-Rich and Contextualized. Paper presented at 31st IEEE International Conference on Robot & Human Interactive Communication, Naples, Italy, August 29 - September 2, 2022. [BibTeX]